What type of content do you primarily create?
A few weeks ago, there was a leak of Apple's hidden AI prompts for their Smart Reply email feature and Memories feature for Apple photos. But most of the attention was given to the prompt around hallucinations: In it, the engineers implored the system, "Do not hallucinate. Do not make up factual information."
If only it were that easy! The instructions were widely panned for being naive—a stark reminder that even the most advanced AI developers grapple with one of AI's biggest challenges: hallucinations.

What are hallucinations?
Originally coined to describe computer vision instances where AI "saw" something that wasn't really there, the term "hallucination" has broadened in meaning over the last few years. Today, it also refers to instances when Large Language Models (LLMs) generate output that strays from real-world facts or deviates from the input prompt. In other words, when your AI pal starts making things up.
These hallucinations come in two flavors: factfulness and faithfulness.
1. Factfulness hallucinations: These happen when LLMs produce factually incorrect information, like claiming that Mount Everest is the tallest mountain in our solar system, or when they craft plausible-sounding falsehoods, such as explaining the history of a fictional scientific discovery.
2. Faithfulness hallucinations: These happen when the LLM partially ignores or deviates from your instructions, leading to inaccuracies. An example might be an LLM that inaccurately summarizes a document or makes a logical error in its reasoning.
Both kinds can result in errors, and they take different techniques to reduce.
Hallucinations are a feature, not a bug
It might be tempting to wish we could get rid of hallucinations completely, but I have bad news on that front. These quirks are not accidental glitches—they are a direct consequence of how LLMs are designed and trained. Hallucinations are not a bug, but a feature deeply embedded in the architecture of Large Language Models (LLMs).
Ironically, the same mechanisms that allow these models to perform incredible tasks, like generating new ideas, summarizing complex documents, crafting creative prose, or explaining complex concepts are also what make them prone to hallucinations.
Recognizing this will give you a better grasp of when and why models hallucinate, helping you identify situations where these errors are most likely to occur. If you want to explore this concept in more depth, check out my introductory article on AI hallucinations. But if you're just after practical tips to reduce hallucinations, keep reading.
Strategies to reduce hallucinations
The good news? Because hallucinations are such a well-known problem, many research teams are actively developing techniques to reduce them and make AI tools more reliable.
Here are some practical tips drawn from this work to help you minimize hallucinations in your AI-assisted tasks.
1. Use the right model
When I was working on this article, I tried to make my AI buddies hallucinate by using almost all of the examples from the papers I read and many homemade examples I cooked up myself. ChatGPT-4o gracefully sidestepped almost all of my hallucination booby traps.
Frontier models like ChatGPT-4o, which are the most advanced and recently developed, tend to hallucinate less than their older counterparts because they’re trained on more up-to-date data and often have more sophisticated architectures. However, bigger isn't always better—some smaller models punch above their weight in tests.
Several rankings exist that track how different models perform in terms of hallucinations, so you can see how they compare.
2. Check your spelling and capitalization
It might seem trivial, but proper spelling and capitalization can significantly impact the AI's response. A misspelled word or incorrect capitalization can change the meaning entirely (think "apple" vs. "Apple"), potentially leading the AI down the wrong path.
I put this to the test with a music video trivia question, comparing capitalized vs. uncapitalized versions. While both sometimes caused hallucinations, the capitalized version was less likely to get the answer wrong after I regenerated the response multiple times.
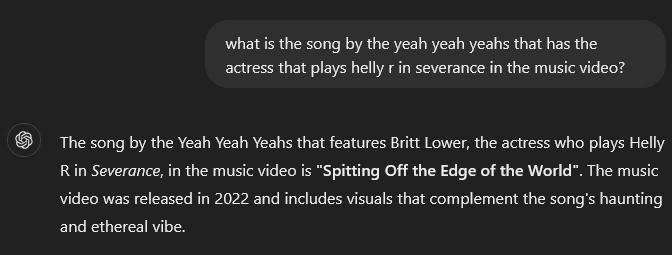
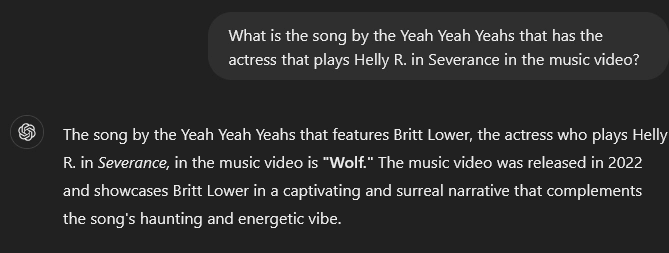
3. Don't force commitment
No, this isn't about which AI you want to spend the rest of your life with. Prompting in a way that forces the model to commit to an answer too soon can unintentionally create hallucinations.
When presented with a yes/no question, AI models often have a tendency to commit to an answer immediately, even if they're not certain. This is where their people-pleasing tendencies kick in: they're trying to give you an answer, even if they're grasping at straws.
One study found that ChatGPT commits to a yes/no answer over 95% of the time, and the answers are usually incorrect and followed by an incorrect explanation.

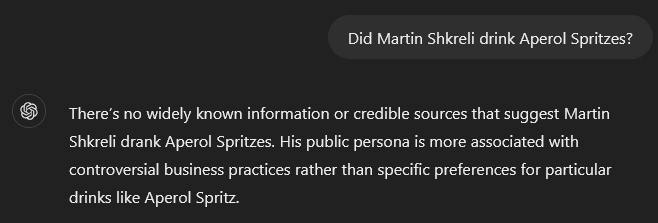
Researchers found that most of the time, LLMs "know" when they have given incorrect information in these contexts. As a result, asking the model a follow-up can identify hallucinations, but they can also snowball or overcommit to incorrect responses. When in doubt, start a new chat window if you think it's snowballing.
It's also a problem when asking the LLM to solve more complex problems. That's because when you ask the AI to solve a multi-step problem in a single step, like determining whether a number is prime, it may commit to an answer before reasoning through it. Since the model’s sequence context only works one way (forward), it can make mistakes.
The silver lining? The technology is improving, and many newer models have started explicitly stating when they're not sure of an answer. Although most current models will avoid this commitment due to their internal instructions, an easy way to fix it is to just add the instruction "Let's think step by step" to your prompt, and then verify its reasoning.
4. Be clear
The clearer and more specific your prompt, the less room there is for the AI to go off on a creative tangent. Vague or ambiguous prompts can lead the AI to make assumptions or fill in gaps with potentially incorrect information or wrong outputs.
5. Ask the AI to browse the web
LLMs are, at their core, sophisticated pattern-recognition and text-prediction machines. They don't possess true understanding or knowledge in the way humans do. A useful way to nudge them closer to that understanding is to lean on the knowledge of humans, such as that found online on high-authority websites and knowledge sources like Wikipedia.
Some AI models, like those used by Perplexity or Bing, can browse the web in real time to find and verify information. To use this strategy, you might have to explicitly ask the AI to search the web for the most up-to-date information on your topic. Be aware, however, that while this method can improve accuracy, it's not infallible—the AI could still misinterpret or incorrectly summarize the information it finds.

6. Retry the prompt
If you're not sure about an answer your AI has given, you can retry the response multiple times to see whether the LLM is consistent with its answer. Better yet, try various LLMs and see if they agree. Different models have different architectures and training sets, so by combining them you can play to the strengths of all of them. Although this doesn't guarantee you're not getting a hallucination, it can alert you to times where the model's response is less stable.
Pro tip: Make sure you try this when you're asking for any kind of strategic recommendation. You might be surprised how different its suggestions can be from one attempt to the next!
Advanced techniques to reduce hallucinations
These strategies can reduce many different kinds of hallucinations, but there are also some advanced techniques if you're still having issues. We've covered a few examples of prompts that improve accuracy as well.
CoT: Chain of Thought
Chain of Thought (CoT) is useful for reasoning through complex problems. This technique improves accuracy by guiding the model through logical steps, helping to avoid the issues discussed earlier. First you prompt the LLM with the question, and then provide a step-by-step guide for the LLM to work through to come up with an answer.
Chain of Thought template:
Problem: [Insert your complex problem here]
Let's approach this step-by-step:
1. First, we need to understand...
2. Next, let's consider...
3. Now, we can calculate...
4. Finally, we can conclude...
Please follow this thought process to solve the problem.
CoVe: Chain of Verification
In Chain-of-Thought, you have to come up with your own intermediate steps, but Chain of Verification's four-step process generates its own. First, the response is generated from the LLM. Next, the LLM plans a suite of verification questions that could be used to analyze whether the response contains errors. The LLM then executes those verifications and uses it to generate a final response.
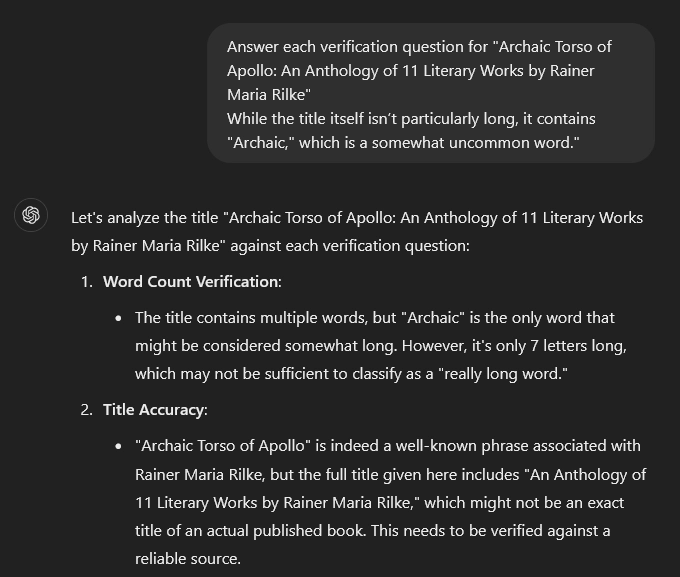
Chain of Verification template:
1. [Original Prompt]
2. For the following Question-Response pair, generate a list of [up to 3] verification questions that will analyze whether there are any mistakes.
[Question-Response pair]
3. (For each verification question, potentially use a new chat window to avoid snowballing) Answer the following question: [Paste verification question].
4. Generate a response to the following question [Original Prompt] given the following results [Verification results]
Pro Tip: Although CoVe works well, I've found that it usually comes up with a lot of questions, and so going through the full process is overkill. In your prompt, you might want to ask for a smaller number of verification questions.
Re2: Re-reading the Question
Re-read the question: it might sound simple, but it’s surprisingly effective. Researchers have found that requiring the LLM to read the question twice can help to reduce hallucinations. Because of their architecture, LLMs process text in a forward-only direction. However, they theorize that by presenting the question twice, this technique helps overcome that limitation, effectively allowing the LLM to understand it in two directions.
Re-reading the Question template:
[Question]
Read the question again: [Question]
Example:
Roger has 5 tennis balls. He buys 2 more cans of tennis balls. Each can has 3 tennis balls. How many tennis balls does he have now?
Read the question again: Roger has 5 tennis balls. He buys 2 more cans of tennis balls. Each can has 3 tennis balls. How many tennis balls does he have now?
The big guns: Retrieval-Augmented Generation (RAG)
If you're still not satisfied with your reduction in hallucinations, there is one more possible tool: Retrieval-Augmented Generation (RAG). RAG is gaining a lot of popularity and is usually used in important contexts where hallucinations absolutely need to be minimized. Think: customer service bots that need to tell you accurate information, or health care bots that need to get symptoms and treatments right.
It has a unique process beyond just the prompt. RAG guides the LLM through a larger process to arm it with the information needed to respond to the user query before responding. First, it retrieves supporting documents or data sources, and then it generates a response based on the retrieved data.
To implement RAG, you can set up a custom bot with your own documents and retrieval system. In your system prompt, you can specifically ask the AI to quote the retrieved document to minimize hallucinations even more. It's a pretty involved process—much more than just prompt wizardry—but if you’re working in a high-stakes area, it might be worth the effort.
Hallucinations, be gone?
Hallucinations are one of the most persistent challenges in the world of AI, but they aren’t insurmountable. There are lots of strategies to use, some simple and some more advanced. But don't forget the motto "Trust, but verify." That goes for all important things you pull out of LLMs, no matter how sophisticated they get.
Psst: If you want to know why hallucinations happen and how to spot them, check out what are AI hallucinations, and why do they happen?
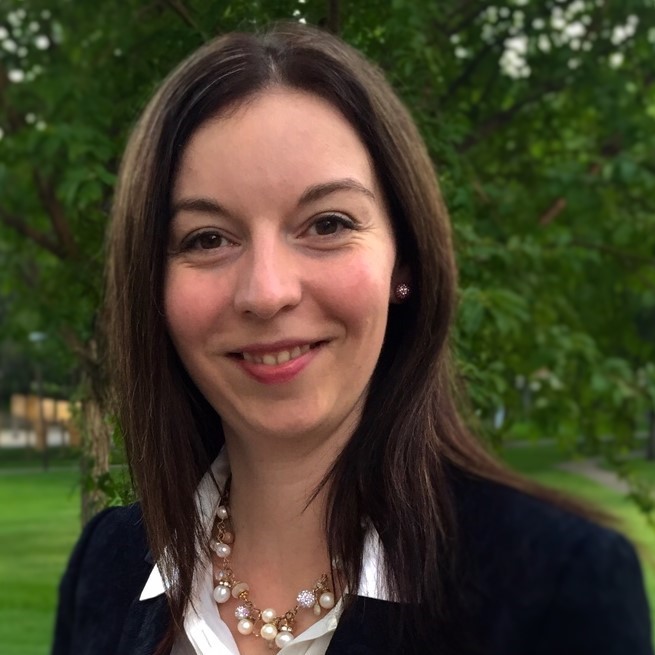