What type of content do you primarily create?
ChatGPT is a confident liar. It delivers completely made-up information with the unwavering certainty of a politician during election season—and that's actually a problem when you need reliable answers.
The AI world calls these fabrications 'hallucinations'—a surprisingly cute term for when an algorithm just invents facts out of digital thin air. The good news? These AI systems are getting better at sticking to reality rather than fiction.
Even better, you can actually reduce these fabrications yourself. Recent research shows that using specific prompting techniques significantly improves ChatGPT's accuracy. These techniques aren't just for fact-checking (which you should absolutely still do) but also enhance strategy development, brainstorming sessions, and data organization.
Here are five research-backed prompting approaches that can make ChatGPT tell you what it actually knows, rather than what it thinks sounds plausible.
1. Few-shot prompting for ChatGPT accuracy
The guiding principle here is simple: show, don't tell. Instead of explaining what you want from ChatGPT, give it a few examples to learn from. This approach significantly improves accuracy by providing clear patterns for the AI to follow.
I've found this technique is pretty versatile, especially for crafting sophisticated categorizations or extracting specific details from longer texts. For instance, I've used it for categorizing online reviews into positive or negative sentiments, identifying main complaints in a review, or categorizing a transcript into topics.
This prompt style is best when you already have examples to work with, but gets annoying fast if you have to come up with examples from scratch before using it. When trying to get accurate ChatGPT responses, the quality of your examples directly impacts the quality of results.
How many examples are enough? It turns out more is not necessarily better. Load up too many examples, and the AI struggles to generalize, leading to errors. It's crucial to find the sweet spot for your specific use case. Usually two to three is a good amount.
Tip: To maximize the effectiveness of this style and reduce ChatGPT hallucinations, clearly delineate the different parts of your prompt—using delimiters or tags works wonders. This helps ensure your questions aren't muddled up with your examples.
Example:
Please generate a podcast episode title for my new episode. Description: Discussing the historical influence of jazz music on modern pop genres.
Please follow the same format as the examples:
Description: An interview with a professional athlete about their training routines and mental preparation. Title: "Peak Performance"
Description: A deep dive into the latest advancements in renewable energy technologies. Title: "Renewable"
Description: Discussion on the impact of social media on teenage mental health. Title: "Antisocial Media"
![]() |
2. Chain-of-thought prompting method
When you ask a LLM to do something, it comes up with an answer for you without explicitly going through intermediate steps to get there. That usually works with simple tasks, but when the question is complex, an LLM skipping the middle steps can struggle to get the best answer, leading to accuracy issues. (I guess they missed the "show your work" mantra we all learned from high school math class.)
Chain-of-thought fixes this problem. It asks the tool to explicitly go through a series of pre-specified reasoning steps before answering.
Researchers have found that chain-of-thought prompts have the potential to make ChatGPT a math and logic whiz, improving accuracy by up to 30% on complex reasoning tasks. Plus, since this prompting style asks the LLM to lay out its thought process, you can easily trace where it might have slipped up.
However, to harness this magic, you need to master the setup, which can be a bit of a chore. First, you have to identify the necessary intermediate steps. You can rely on your own knowledge here, or if you're stumped, ask the AI for help. This might get frustrating, especially when the steps aren't clear cut, but often, the AI is surprisingly good at proposing useful starting points.
I've found chain-of-thought really valuable for strategy and planning questions, where I can ask ChatGPT to work through options using a specific rubric before drawing a conclusion. By tweaking the criteria, you can shift which option appears most favorable, making it a powerful tool for weighing different factors and getting reliable responses.
Example:
I have three ideas for a podcast episode. Please prioritize them. First, consider the potential engagement and reach, Second, consider the alignment with my current content. Next, make a recommendation for which one to produce. [Third, consider whether engaging multimedia elements could be included.]
Idea 1: Dada Songs: Music, Nonsense, Individuality
Idea 2: Like Clockwork: Time, Schedules, Rhythm
Idea 3: Lies, Noble & Otherwise: Knowledge, Deception, Truth
Here are my current episodes:
1. All Life on Earth: Growth, Survival, Evolution. In the mid-1920s, Josephine Salmons discovered a unique fossilized skull in South Africa, leading to the identification of a new hominid species, Australopithecus africanus, by her professor, Raymond Dart. This find shifted the scientific consensus towards Africa as the "Cradle of Humankind" and sparked debates about human origins. The episode "All Life on Earth" also explores broader questions of intelligence and uniqueness across species, examining traits shared between humans and other life forms, and delving into how technologies like machine learning might blur these distinctions further.
2. Feast & Famine: Cooking, Agriculture, Gastronomy. The podcast episode "Feast and Famine" examines the significant role of food in human culture, noting how cooking transformed human society by enabling the consumption of a wider range of foods and fostering communal meals that strengthened social bonds and traditions. It also explores the impact of agriculture in supporting larger populations and delves into the conceptual realm of machines mimicking eating, like the 18th-century Digesting Duck, posing questions about the uniqueness of social eating in human development.
![]() |
3. Rephrase and respond prompting technique
Ever assigned a task to someone who nodded enthusiastically as you explained but delivered something that made you think they weren't listening? Turns out the same kind of disconnect can happen when you're working with LLMs, often leading to ChatGPT accuracy issues and hallucinations.
Fortunately, there's a handy technique to bridge this gap: "Rephrase and respond." This prompt strategy helps clarify any ambiguities by having the AI rephrase your original prompt before it begins to answer.
Here's the gist: When we explain something, we often leave out our implicit assumptions—things so obvious to us that we don't think to mention them. But what's obvious to us might not be so clear to an AI. Rephrase and Respond can help make ChatGPT more accurate. This prompt technique asks the LLMs to rephrase the input question before responding to it.
Turns out, this is a pretty simple one to implement. All you need to do is add an additional line to your prompt. The paper suggests four variations you can use:
- Reword and elaborate on the inquiry, then provide an answer.
- Reframe the question with additional context and detail, then provide an answer.
- Modify the original question for clarity and detail, then offer an answer
- Restate and elaborate on the inquiry before proceeding with a response.
Choose whichever you like best; their performance is about equal.
Once the LLM rephrases your prompt, it'll show you whether something was unclear so you can correct it.
In my tests, this method worked best with very short prompts, but sometimes ignored the instruction when I got too wordy. To get it to work even better, I put my original prompt in quotes before adding the Rephrase and Respond instruction to the end.
Tip: Researchers found that this resulted in a significant bump in accuracy (approximately 15-20% improvement), even over and above Chain-of-Thought prompting— though you can combine it with Chain-of-Thought prompting to improve your ChatGPT accuracy even more.
4. SimToM prompting for better accuracy
In essence, SimToM prompting is a way to get ChatGPT to take a human's perspective, enhancing its reasoning capabilities and reducing hallucinations. It's rooted in the Simulation Theory and the psychology of Theory of Mind. No PhD required here—we'll break it down.
Theory of Mind is our ability to understand that others have their own beliefs, desires, and intentions, distinct from our own. We manage this through "perspective-taking," where we imagine stepping into someone else's shoes, and "question-answering," where we interpret and reason as if we were that person. This might sound straightforward, especially if you're the type who always figures out who committed the crime in a mystery novel, but it's a tough nut for even the most advanced LLMs to crack.
Humans are pretty adept at the "What are they thinking?" game, and the SimToM prompt method attempts to replicate those innate skills with a two-step prompt. First, you prompt the LLM to outline what's known to a specific character. Next, you have it adopt that character's perspective to answer a question.
SimToM is a great prompt to use for storytelling, to dig into motivations or emotional reactions. Where I think this prompt style would truly be magic is to help figure out plot points for a story and understand how the various characters might act.
But the characters don't have to be fictional; you can also use SimToM to explore audience or customer personas, for example. I found it particularly handy to use this method to simulate a hypothetical customer journey.
Template
Prompt 1: Perspective taking
The following is a sequence of events:
{story}
Which events does {character_name} know
about?
Prompt 2: Question-Answering
{story from character_name's perspective from Prompt 1}
Answer the following question:
{question}
Template
Prompt 1: Perspective taking
Mary is a professor at the University of Somewhere. She has been teaching economics for 20 years. Recently, she has noticed that the overall quality of the assignments submitted has improved, but test scores have dropped in her macroeconomics course. Students have been using ChatGPT to complete their assignments. They are also spending less time on the material week over week.
What does Mary know?
Prompt 2: Question-Answering
What resources does Mary need in order to support her teaching in this course?
5. Step-back prompting approach
Ever find yourself pausing for a deep breath before jumping into a complex problem? That's not just you being cautious—it's a smart strategy to improve accuracy! Step-back prompting takes advantage of this natural tendency to think before you act by applying it directly to how we interact with language models like ChatGPT.
Step-back prompting does this by tapping the strategy humans use when faced with a complex problem: considering the broader context first. This allows us to draw on high-level concepts and first principles to craft our answers, significantly reducing ChatGPT hallucinations. An example might be recalling the laws of physics before tackling a specific question about temperature.
Here's how the process works. You first ask the LLM a "step-back question" to set the stage, then use that broader perspective to inform the answer to your main query. The step-back question is generally more abstract and simpler than your targeted question. It often involves generating a broader list of options or a summary, setting the stage for a more focused follow-up.
Although it was initially set up to try to pull correct information out of longer text (and it is useful for that), I find this more useful as a formalization of what many of us already do when brainstorming with AI: start by asking the LLM for a wide range of options, then refine these choices based on more specific criteria. Essentially, you go wide first (step-back) to establish the context, then zero in (Question) on what you need. For example, you might consider who the most popular musicians were in 1988 before deciding whose musical contributions best fit with a specific narrative arc.
Example:
Step-back question: Who were the spouses of Anna Karina?
Original question: Who was the spouse of Anna Karina from 1968 to 1974?
Step-back question: What major tech developments have happened in the last two years?
Original question: What tech developments should we cover in our next podcast season?
![]() |
![]() |
Why ChatGPT hallucinates and how to address it
ChatGPT, like other large language models (LLMs), can produce factually incorrect statements known as hallucinations. They often occur because of data quality issues or suboptimal training objectives demonstrated by extensive research. Additionally, inference-time decoding strategies can inadvertently generate content that deviates from factual data according to published findings. Mitigating these errors involves verifying AI outputs through cross-referencing, expert review, or reliability scoring to identify possible discrepancies. In many cases, encouraging ChatGPT to use chain-of-thought reasoning or rephrase-and-respond prompts can address some of the root causes of hallucinations without requiring specialized technical interventions. Still, advanced domain-specific data training remains particularly useful for scenarios where factual accuracy is paramount as shown in retrieval-augmented frameworks.
How these prompts improve ChatGPT accuracy
I'm always excited when I get to try and test new advanced prompting methods because the evolution of these techniques reflects our growing understanding of how AI "thinks" and our continuing quest to make ChatGPT more accurate and adaptable to our needs.
While I won't be giving up my favorite advanced style, the Flipped Interaction Pattern, I've started integrating these other approaches to my workflow, especially in my brainstorming and strategy work. By combining these prompt engineering techniques, you can dramatically improve ChatGPT accuracy and get more reliable, trustworthy responses. I hope you will too.
FAQs
How can I ensure ChatGPT's responses are accurate?
Start by using structured prompts, like chain-of-thought or rephrase-and-respond, to reduce confusion. Always cross-reference AI outputs with reliable sources, especially for specialized topics. According to peer-reviewed research, employing a reliability scoring or an expert-led validation process can further reduce errors. Lastly, refine your prompts continuously based on feedback to keep improving results.
What is hallucination in AI?
Hallucination refers to instances where AI models produce made-up or inaccurate information with unwavering certainty. One study shows that data quality issues and suboptimal training methods often contribute to these errors. This becomes especially problematic when users rely on AI for domain-specific or high-stakes information. Taking steps such as verifying with human experts can mitigate the impact of hallucinations.
What if ChatGPT provides incorrect domain-specific data?
Domain-specific inaccuracies often arise when ChatGPT has insufficient training data in that area. Researchers suggest enhancing knowledge with retrieval-augmented methods or domain-targeted datasets. This helps the AI generate more precise information for specialized fields like medicine or finance. Double-checking ChatGPT's output with reputable sources and subject-matter experts remains essential for accuracy.
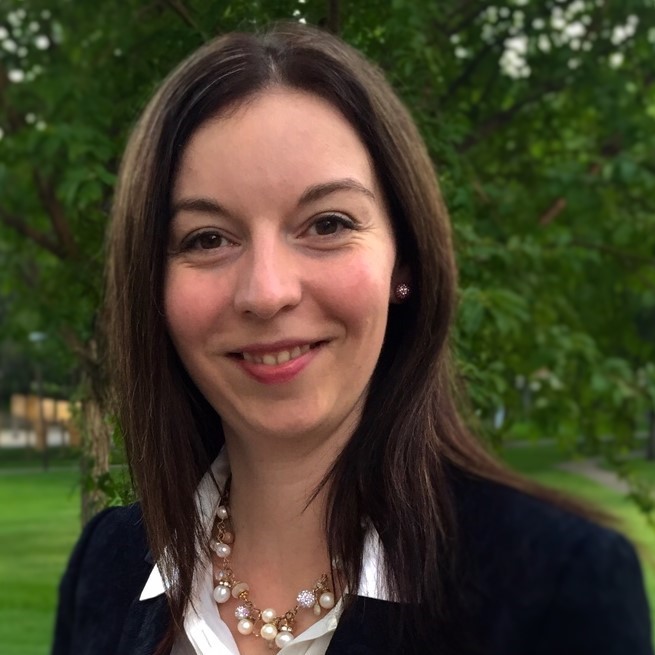